INTERNATIONAL
ADMISSIONS
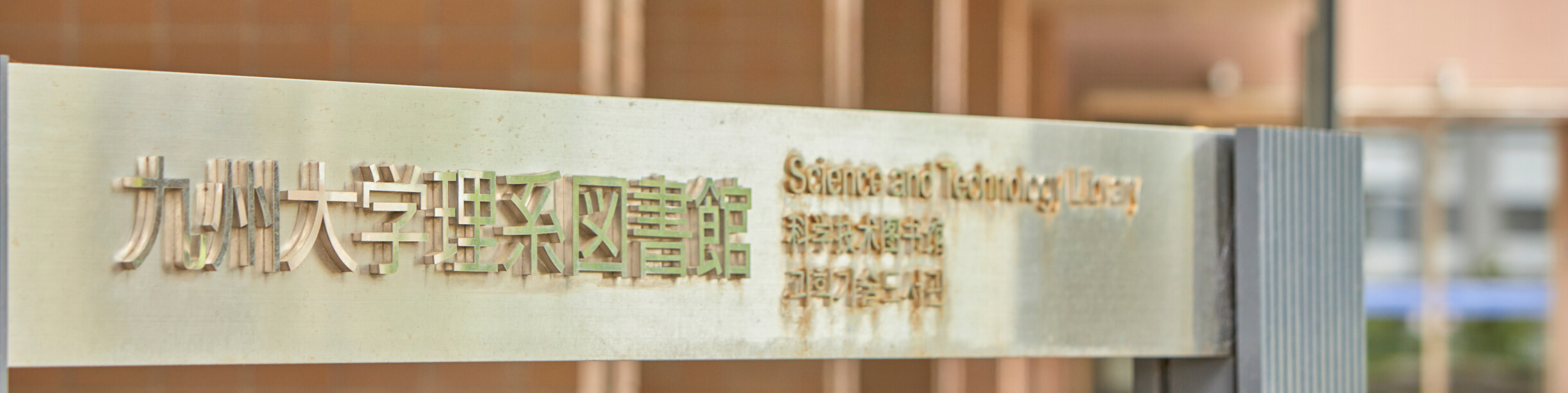
Welcome. The purpose of this webpage is to provide information to the students who are interested in continuing their studies in mathematics at the graduate school of mathematics at Kyushu University.
General Information about Study abroad at Kyushu university
Kyushu university is one of 11 Research universities (RU11) in Japan founded 1911. The campus is new and modern, located in the suburbs of Fukuoka city. For general information for foreign students who wish to study at Kyushu university, we refer the following websites.
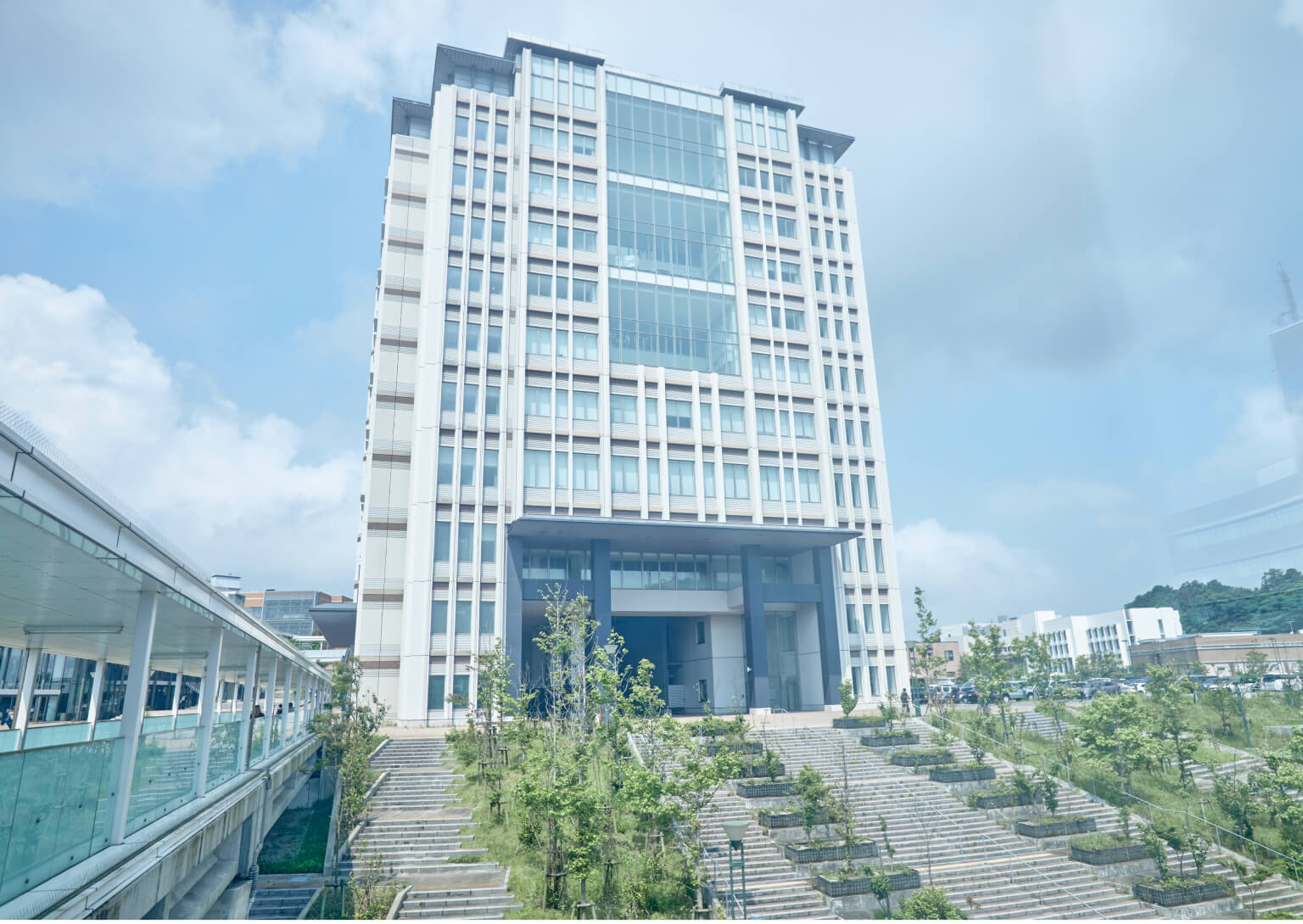
Briefly about our graduate school
The Graduate School of Mathematics at Kyushu University has over 70 researchers, forms the top league for mathematical research in Japan, together with mathematics institutes in The university of Tokyo, Kyoto University, and Nagoya University, among others. One of the notable features of our school is the wide range of mathematical research conducted, spanning from fundamental research in cutting-edge pure mathematics to applied mathematics with a focus on applications in various industries.
Most of the faculty members are internationally recognized researchers in various fields of mathematics and willing to accept foreign students in Ph.D course. We hope you will find a good supervisor of your study among our faculty members. The institute provide excellent modern environments for students studying mathematics (sufficient spaces, good Library with rich collections of Literature, up-dated Computer facilities, etc).
As for most universities in Japan, for graduate studies, your choice of a supervisor is typically the most important factor. This holds true for international students as well. If you have a desire to continue your studies in mathematics at Kyushu University, we strongly recommend that you locate and reach out to a faculty member in our Graduate School of Mathematics. By demonstrating your abilities and your determination to pursue further study, they will provide their assistance and support.
If prospective students are not familiar with the desired faculty member who will be their supervisor, it is advisable to first contact our Pre-Admissions Desk to verify your identity and documents before reaching out to him or her. This will help ensure that your emails reach the faculty member accurately.
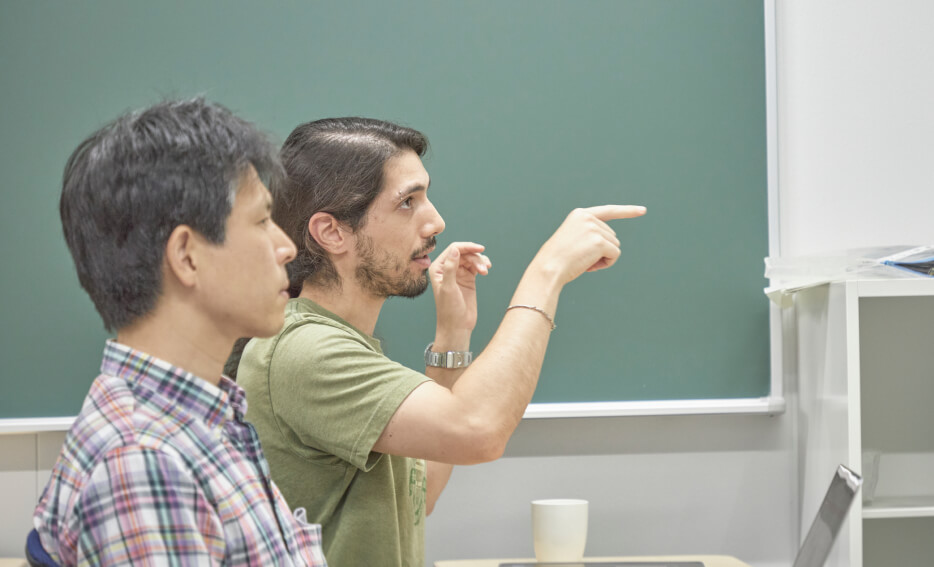
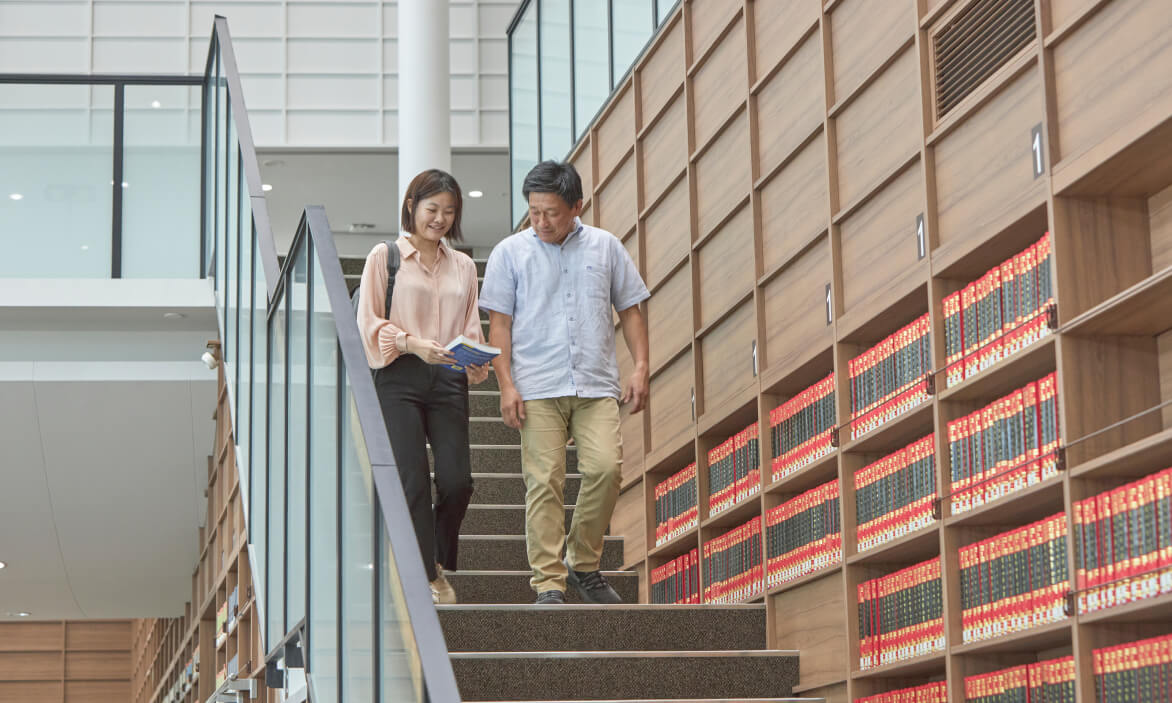
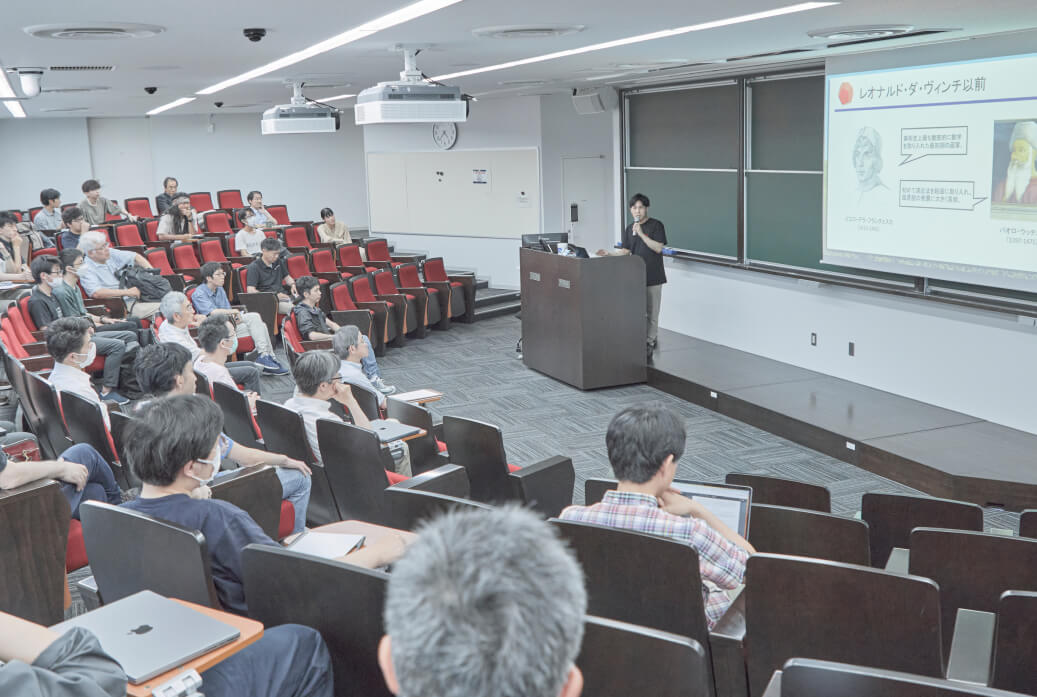
Admissions
In our graduate school of mathematics, we offer both a Master’s course and a Doctoral course. The Master’s course typically takes two years to complete, while the Doctoral course typically takes three years (after obtaining a Master’s degree). Admissions are available for both April and October intakes, and the application procedures usually begin two to four months prior to the start date. We recommend that applicants start preparing for admission at least six months in advance.
For international students, there are also two options to apply:
1) Special Screening of International Students (Master, Doctor)
2) Admission for International Course (Master, Doctor)
The second option is specially designed for international students who are not proficient in the Japanese language. All the lectures and seminars are conducted in English.
NOTE: For CSC(China Scholarship Council) Applicants, we provide a special screening a half-year prior to the admission. (See below.)
The selection process consists of a document screening and an interview, which can be conducted either online or in person. The final decision regarding acceptance or rejection is based on our assessments to your applications, as well as the feedback provided by the faculty advisor.
For details, we refer the guidelines of each examination, which will be posted as a news in this website. (See the bottom of this page.)
Guidelines for Admissions
The application guidelines will be published in the NEWS section at the bottom of this page according to the schedule provided in the list below. The guidelines for the upcoming year are expected to remain largely unchanged from those of the previous year, with only minor adjustments made to dates or other details. If the specific guideline you are looking for has not been published when you visit this site, referring to the guidelines of the previous year will be helpful.
Schedules for Admission
Type of
AdmissionsDegree Type
Admission
PeriodRelease of
GuidelineApplication
PeriodSpecial
ScreeningM,D
Spring
(April)Early November
Early January
D
Autumn
(October)Mid-May
Mid-July
International
CourseM,D
Autumn
(October)Mid-January
Early April
Special
Screening for
CSCD
Autumn
(October)Early November
Early December
More Information
The tuition of graduate school is around 550,000 yen. Doctoral course students will be hired as RA and the yearly salary is almost equivalent to the tuition.
The average accommodation rate is 45,000-60,000 yen/month (off-campus) or 21,000-30,000 yen/month (dorm, on-Campus, not including utilities). The living expenses will be less than 2Million yen per year. For accommodation, the following webpage will be useful.
There are a few scholarships and programs in the Graduate School of Mathematics or in Kyushu University where Doctoral students are provided by living and research expenses (2Million yen/year).
NOTE: The screening for these courses are taken place only after you enter in the graduate schools at Kyushu University.
NEWS
2025.5.27
Admission of Doctoral Course in October, 2025
2025.5.21
Announcement of successful applicants
2025.1.16
International Programs of Graduate School of Mathematics
2024.12.17
Application Guidelines for Research Students
2024.10.31
Special Screening of International Students for Admission in April, 2025